728x90
반응형
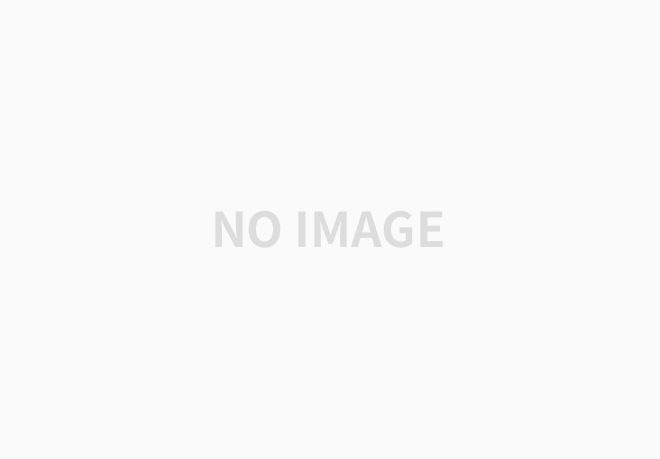
AI 학습 과정 설명
AI 모델을 통해 사용자 맞춤형 이력서를 생성하는 과정은 데이터 수집, 전처리, 모델 학습, 그리고 텍스트 생성을 포함한 일련의 단계로 구성됩니다. 아래는 이러한 과정에 대한 구체적인 설명입니다.
+--------------+ +--------------+
| 사용자 입력 | ----> | AI 모델 |
+--------------+ +--------------+
| |
| API 호출 (POST 요청) |
| ----------------------> |
| |
v v
+--------------+ +--------------+
| 데이터 처리 | <---- | AI 모델 결과 |
| (FastAPI) | +--------------+
+--------------+
|
v
+--------------+
| 이력서 생성 |
| (LangChain) |
+--------------+
|
v
+--------------+
| 결과 반환 및 표시 |
+--------------+
1. 데이터 수집 및 전처리
사용자 입력 데이터를 학습 데이터로 변환
def generate_finetune_data(user_info):
training_data = []
for section_name, section_text in user_info.items():
if section_text:
training_data.append({
"prompt": f"{section_name}: ",
"completion": section_text.strip()
})
return training_data
- 목적: 사용자로부터 입력된 이력서 섹션 데이터를 GPT-3 모델이 이해할 수 있는 형식으로 변환.
- 작업: 사용자 입력 데이터를 학습 데이터 형식(JSONL 파일)으로 변환.
- 출력: training_data 리스트에는 각 이력서 섹션의 이름과 내용이 포함됩니다.
2. 모델 학습 (Fine-Tuning)
GPT-3 모델을 사용자 데이터로 Fine-Tuning
def fine_tune_model(training_data):
with open("training_data.jsonl", "w") as file:
for item in training_data:
file.write(json.dumps(item) + "\\n")
response = openai.File.create(
file=open("training_data.jsonl"),
purpose='fine-tune'
)
file_id = response['id']
fine_tune_response = openai.FineTune.create(
training_file=file_id,
model="davinci",
n_epochs=4
)
return fine_tune_response
- 목적: GPT-3 모델을 사용자 입력 데이터를 사용하여 Fine-Tuning.
- 작업:
- 사용자 데이터를 포함하는 training_data.jsonl 파일 생성.
- OpenAI API를 사용하여 파일을 업로드하고 Fine-Tuning 시작.
- 여러 Epoch 동안 모델 학습을 진행하여 모델이 사용자 데이터를 기반으로 텍스트를 생성하도록 최적화.
- 출력: Fine-Tuning 완료 후, 학습된 모델 정보(fine_tune_response) 반환.
3. 텍스트 생성
학습된 모델을 사용하여 이력서 섹션 텍스트 생성
def generate_custom_resume(prompt):
template = PromptTemplate(
input_variables=["user_info"],
template="You are an expert resume writer.
Given the following user information,
create a resume.
\\nUser Information:\\n{user_info}\\nResume:\\n"
)
llm_chain = LLMChain(prompt_template=template,
llm=OpenAI(model="davinci"))
response = llm_chain.run(user_info=prompt)
return response
- 목적: Fine-Tuning된 GPT-3 모델을 사용하여 사용자 맞춤형 이력서 섹션 텍스트 생성.
- 작업:
- LangChain의 PromptTemplate을 사용하여 텍스트 생성 템플릿 설정.
- LLMChain을 통해 GPT-3 모델과 템플릿을 연동.
- 사용자 데이터를 입력으로 받아 모델이 텍스트를 생성하도록 요청.
- 출력: 생성된 이력서 섹션 텍스트.
4. ResumeAI 클래스 정의
이력서 생성을 총괄하는 클래스
class ResumeAI:
def __init__(self, user_info):
self.user_info = user_info
self.training_data = generate_finetune_data(self.user_info)
self.fine_tune_response = fine_tune_model(self.training_data)
self.sections = {
"growth_background": "",
"education": "",
"projects": "",
"strengths_and_weaknesses": "",
"certifications": "",
"job_position": "",
"job_description": ""
}
def generate_resume(self):
resume = ""
for section_name in self.sections.keys():
prompt = f"{section_name}: {self.user_info[section_name]}"
section_text = generate_custom_resume(prompt)
resume += f"### {section_name.replace('_', ' ').title()}\\n{section_text}\\n\\n"
return resume
def update_section(self, section_name, section_text):
self.sections[section_name] = section_text
def clear_sections(self):
for section_name in self.sections:
self.sections[section_name] = ""
- 목적: 사용자 입력 데이터를 기반으로 Fine-Tuning과 텍스트 생성을 관리.
- 작업:
- 사용자 데이터를 받아 generate_finetune_data와 fine_tune_model을 통해 모델 학습을 준비하고 실행.
- generate_resume 메서드를 통해 각 이력서 섹션에 대해 텍스트를 생성.
- 이력서 섹션 업데이트 및 초기화 기능 제공.
- 출력: 최종 생성된 이력서 텍스트.
AI 학습 과정 요약
- 데이터 수집 및 전처리:
- 사용자 입력 데이터를 JSONL 형식으로 변환하여 모델 학습 데이터로 준비.
- 모델 학습 (Fine-Tuning):
- OpenAI API를 통해 GPT-3 모델을 사용자 데이터로 Fine-Tuning.
- Fine-Tuning 과정에서 여러 Epoch 동안 모델이 사용자 데이터를 학습하여 최적화.
- 텍스트 생성:
- Fine-Tuning된 모델을 사용하여 사용자 맞춤형 이력서 섹션 텍스트 생성.
- LangChain을 통해 템플릿 기반 텍스트 생성 작업 수행.
이러한 과정을 통해 AI 모델이 사용자 데이터를 효과적으로 학습하고, 이를 기반으로 고유한 이력서를 생성할 수 있습니다.
반응형
'LLM(Open AI)' 카테고리의 다른 글
Asterisk PBK 란? 간단한 설명/설정과 구조 (2) | 2024.11.04 |
---|---|
클로드 Claud AI 유료 결제 후기 (2) | 2024.09.30 |
포트폴리오 - LLM 파인튜닝 프로젝트 설명 (2) | 2024.06.03 |
[LLM] 프로젝트를 위한 Open AI API Key 무료 발급 방법 (1) | 2024.05.23 |
LLM (Large Language Model) 대규모 언어(라마인덱스, 랭체인) 간단 (0) | 2024.04.03 |